International experience21.12.2022
Using digital technologies to monitor and predict the health of renewable energy production assets
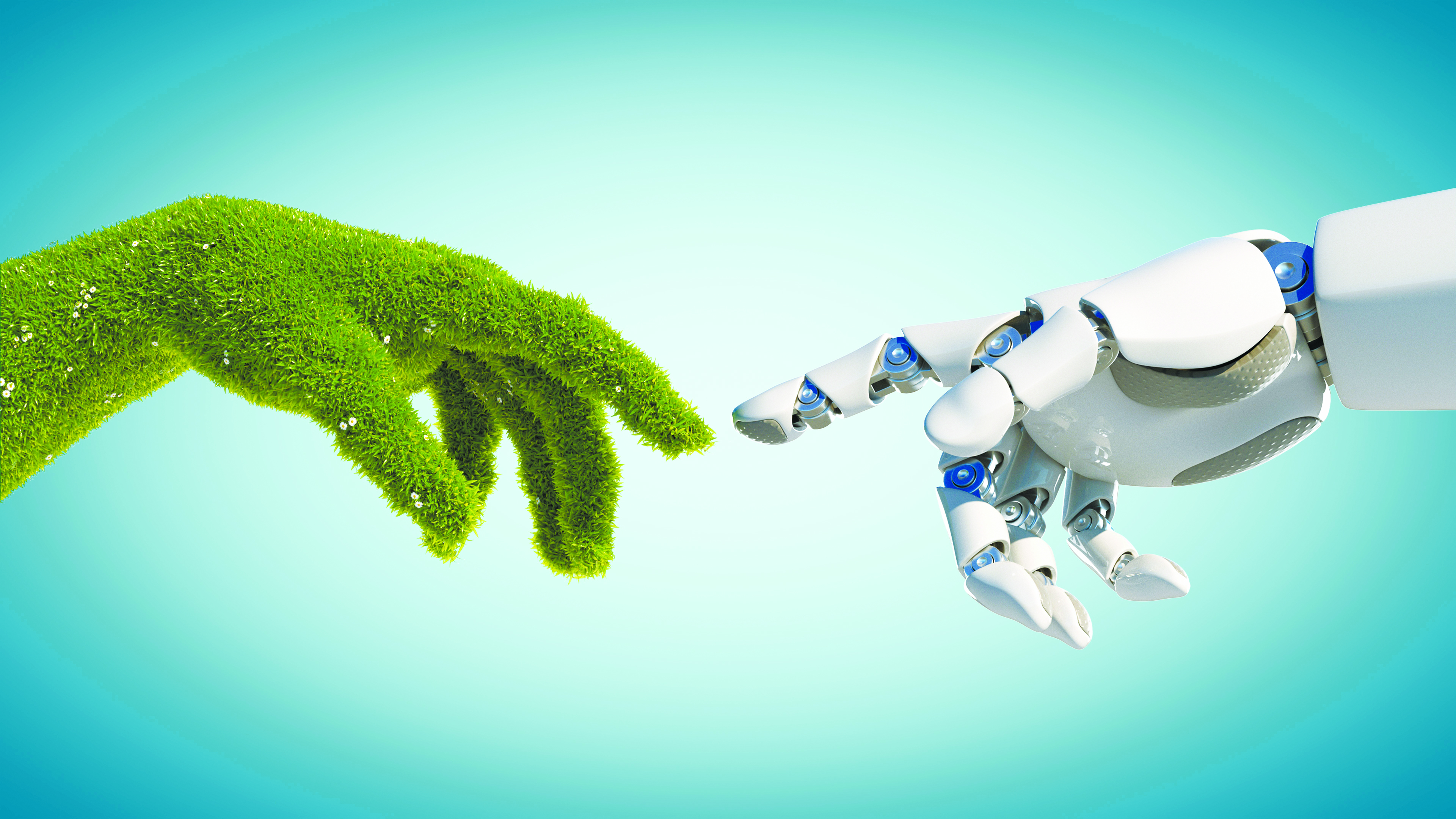
Kazakhstan is seeking to rehaul its current energy infrastructure to make it cleaner and more efficient. This will see the country move away from its reliance on domestic gas, oil and coal resource as it moves towards its goal of supplying 50% of its energy from renewables by 2050. Hand in hand with this will be the opportunities that will arise in the operation and maintenance of these renewable energies. Below, we look at different digital solutions currently available on the market that enable green energy producers, specifically wind energy producers, to prevent, maintain and repair their assets in the most efficient ways possible.
INDUSTRY INSIGHTS
The renewable energy industry faces various challenges, such as competition with conventional energy sources on a cost basis due to high operation and maintenance expenses (O&M). As renewable energies have become more competitive with conventional sources, competition between renewable energy operators increased. With the right strategy, digital technologies help to counteract O&M challenges, give renewable energy operators competitive advantages, and increase their contribution to net-zero emissions.
The sector has made strides in its approach to machine maintenance by moving away from the traditional practice of run-to-failure maintenance which involves “fixing it when it fails”. Currently, many operators use a preventive maintenance strategy which instead favours periodically scheduled maintenance and repair work. Also in use, is predictive maintenance. Predictive maintenance, as suggested by the name, involves knowing when an asset or component will fail, how will it fail, and what will be the root cause of the failure. Going up another level, in prescriptive maintenance, the turbine then also tells the operator how to avoid the predicted failure.
Together, these strategies prevent wind turbine owners, operators, and maintenance providers, from spending resources unnecessarily on the maintenance of their assets.
Based on estimates from European markets such as Germany, the UK and Denmark, O&M costs are estimated to be between 1.2 c€ and 1.5 c€ per kWh of wind power produced, over the total lifetime of a turbine. This translates to 20-30% of the Levelised Cost of Energy. For U.S. markets, O&M costs are usually lower (estimated at $0.01 per kWh).
The cost savings for maintenance and labour can be extensive. Data from the U.S. and Europe suggest the repair and maintenance portion to be between 46% and 57% of overall O&M costs. A 20% reduction in this portion would yield annual cost savings of over $11,000 for a 2.5-MW turbine and about $34,000 for a 7.5-MW turbine. Limiting O&M expenses is a high priority for the wind power sector. Nowadays, given the access to vast amounts of data, the wind farm operators can use machine learning to turn under-utilized sensor data to lower overall turbine O&M costs.
The reduction in O&M spending depends on the use cases identified, available capabilities and the resultant solution developed. For instance, solely Al-focused companies usually expect to see a 3%-5% decrease in O&M costs. Companies that combine industry knowledge, machine learning and reliability modelling see this figure jump to30%- 50%.
Further to this, with the arrival of digitalisation, the industrial loT (lloT), and artificial intelligence (Al), more companies are moving towards getting value out of their assets’ data. This promotes a future where companies use predictive and prescriptive maintenance strategies. To make this possible, solutions should be user-friendly tools using cutting- edge technologies such as digital twins.
DIGITAL TWIN TECHNOLOGY
A digital twin is the digital representation of a physical object or system based on the data that is emitted from the same physical object or system. Digital Twin technology has a huge impact on the product development processes involving engineering knowledge. Digital twins are mainly used to predict various outcomes in different scenarios. As a result, the technology generates great value for businesses that put them to good use.
Most modem renewable energy assets are equipped with SCADA-systems, generating a huge amount of information that can usually be obtained free of any additional cost. Maintenance platforms can use these already existing sensors and data sources to feed data into the digital twin, thus creating a digital copy of the asset that can be remotely used for analysis and predictions. This is one of the greatest strengths that comes with the digitalisation of maintenance operations as it reduces the impact of unplanned outages.
Digital twins ultimately help end-users to better predict, and therefore prevent, future failures while improving performance significantly.
One way in which maintenance developers are utilising digital twins is through the development of holistic dashboards. These dashboards visualise data and boast several modules designed to bring the activities of renewables to the next level.
Comprehensive solutions that are currently on the market offer various modules on lloT-platforms.
Clients can choose which modules fit their need and subscribe to these based on a monthly fee (SaaS). This fee is usually dependent on client requirements, the features and the duration of the maintenance plan booked. Below we look at two key examples of solutions that maintenance platforms seek to offer.
PREDICTIVE MAINTENANCE
A predictive maintenance module is centred around failure prediction. This solution generally provides detailed information on the assets and their components’ health status, remaining lifetime, performance, and operating conditions. In this module, the information is often split into three levels of detail to allow clients to easily access maintenance details as and when they are required.
For instance, the first level aims to provide a high-level overview of all the wind farms which the clients have connected to their maintenance platform. At this level, wind farm assets display KPIs, predicted failures, and malfunctions in real-time. The second level usually offers a more detailed view-allowing the user to zoom closer into the asset data. In this view, information concerning the health status of the turbines, remaining lifetime, performance, and operating conditions should be available at any point in time. The last layer will aim to provide an in-depth, lower-level view of one of the main components. This showcases current and future health statuses, the root cause of any current or future malfunctions, and actionable O&M insights that allow the client to solve any situation that may have developed.
Such failure prediction modules use an automated predictive engine. These engines represent a complex software application that is able to predict wind turbine failures. Some market solutions do so up to a year before they actually occur. This module is usually developed in conjunction with a health index to enrich the data and which showcases the overall operational efficiency of turbine components. Additionally, advanced digital twins provide a detailed estimation of the remaining useful lifetime value of turbines and the recommended maintenance actions. These actions are usually made visible through the various layers of the dashboard as well as a combined overview.
EFFICIENCY UTILISATION
Underperformance detection should be a second target of operations and maintenance solutions, as remedying such issues boosts efficiency massively. ANNEA can offer rich insights into various aspects of the turbine operation, for instance identifying where the most power is lost and the reasons for this loss.
an aggregated view of holistic underperformance activity to be viewed in real-time on wind farm level, considering the wind farm layout and wake effects. This information offers a general but useful showcase of the power production, power loss, the detected underperformance, and its cause. Additionally, it provides actionable suggestions on how to improve performance and offers an understanding of the performance related state of the wind turbine. The second layer usually provides an overview of the turbine’s underperformance, highlighting the relationship between energy loss, loss of revenue, and total energy loss. Clients are able to view the efficiency of the turbine and the costs associated with underperformance and inefficiencies.
Maintenance platforms are also able to monitor turbine components to detect underperformance and provide recommendations. That is when data-driven underperformance modules detect the root cause and provide recommendations on how best to counteract the problem. By following the recommendation, clients can boost the performance of their assets by improving energy production and minimising revenue loss.
CONTRIBUTION TO KAZAKHSTAN’S GREEN ENERGY PLAN
In line with the goal of the Qazaq Green Association uniting renewable energy players to create favourable conditions for industry development, maintenance platforms are keen to offer the association members their expertise and unique solutions to further pursue their cause.
Combining digital twin technology with different modelling techniques enables green energy producers to predict failures up to 12 months ahead on a component level with 99.9% accuracy, without installing additional hardware. With the right strategy, cutting-edge technology from maintenance developers and their counterparts within wind operations - we can overcome maintenance challenges often associate with renewable and contribute to achieving net-zero emissions.
Platforms such as the one by ANNEA utilise predictive maintenance, machine learning and reliability modelling to enable increased reliability, minimise unplanned downtime and offer an extended lifetime whilst simultaneously optimising performance.
Get in touch with an ANNEA representative to understand how your maintenance systems can be utilised to best improve efficiency: www.annea.ai
IRENA accepting renewable energy project proposals in Central Asia until August 15
Astana to host Electronica Expo Kazakhstan Electronics Exhibition
WB gives rundown of Azerbaijan's green energy grid volume prospects
US solar sets new records as renewables nearly match natural gas – EIA
‘Wings’ on poles: Bill Gates-backed breakthrough wind turbine facility breaks ground
Perovskite tandem solar cell achieves new efficiency record
Kazakhstan and China endorse draft SCO joint statement on sustainable energy development
Innovative research on organic solar cells for space applications
Kazakhstan and Uzbekistan drive green energy progress in Central Asia
KazMunayGas launches pilot green hydrogen project in Atyrau
How private homeowners in Kazakhstan can make money from solar panels
14 countries are investing in Kazakhstan's renewable energy sector through auctions
How green hydrogen could transform Kazakhstan’s energy sector
IKEA offers ready-to-use solar power systems for balconies
Adani commissions India’s first off-grid green hydrogen pilot plant
Tajikistan unveils green energy roadmap at international conference in Dushanbe
Hydropower projects selected through KOREM Auction in Kazakhstan
EU reaches political deal to simplify CBAM
New research could unlock the potential of bladeless wind turbines
Uzbekistan to launch 16 renewable energy facilities by the end of 2025